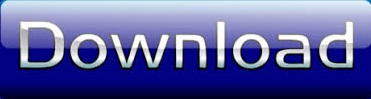
To tackle this challenge, a number of explanation methods have been proposed to expose the inner workings of a DNN underlying its decisions. From a practical and ethical point of view, this is one of the major roadblocks in translating cutting-edge machine learning research into meaningful clinical tools. However, DNNs are often referred to as black boxes since their decision mechanisms are not transparent enough for clinicians to interpret and trust them. Trained on various diagnostic tasks in imaging-based specialties of medicine, they have been shown to achieve physician-level accuracy.
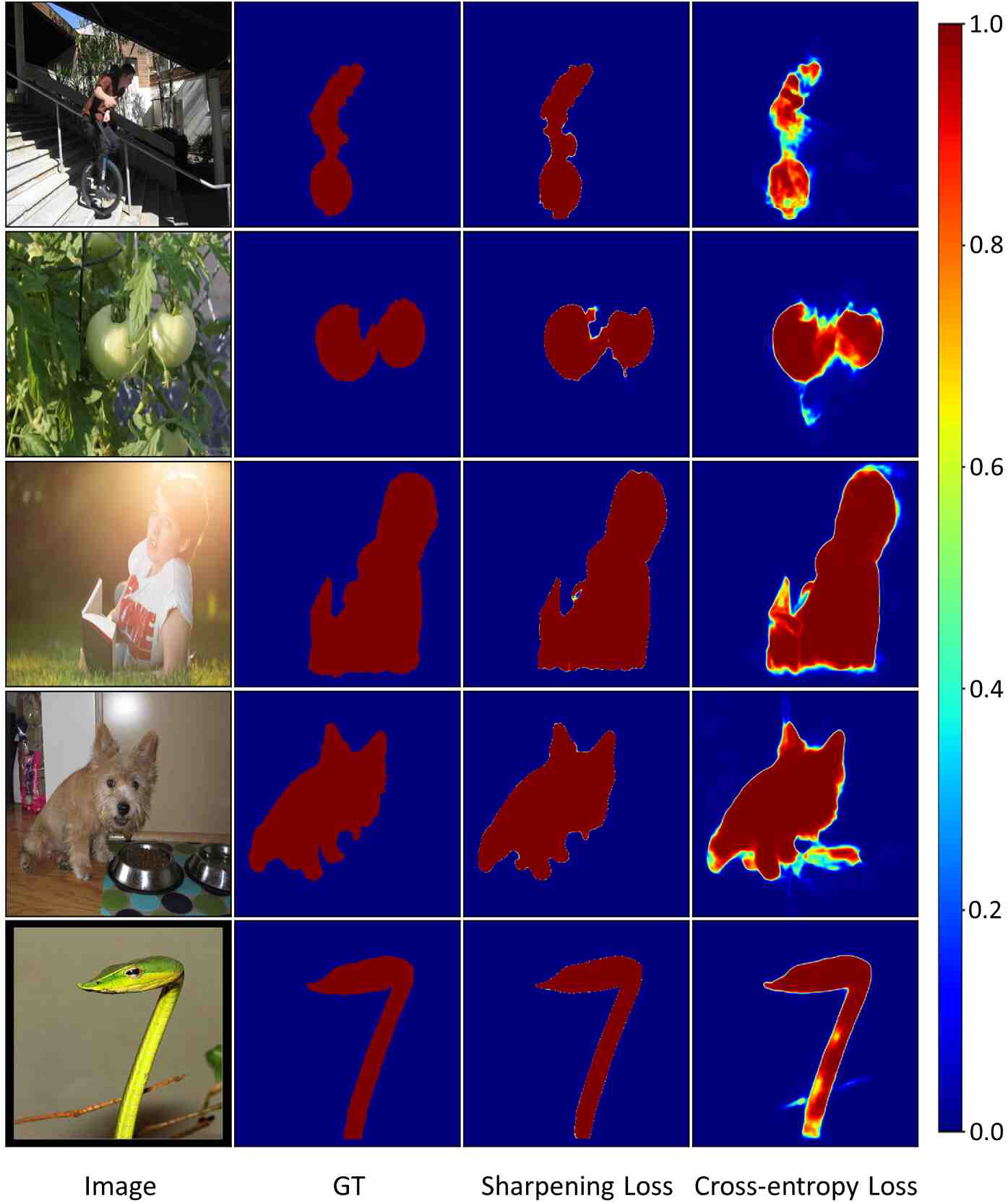
Guided Backprop showed consistently good performance across disease scenarios and DNN architectures, suggesting that it provides a suitable starting point for explaining the decisions of DNNs on retinal images.ĭeep neural networks (DNNs) have become increasingly popular in medical image analysis. We found the choice of DNN architecture and explanation method to significantly influence the quality of saliency maps. Then, we systematically validated saliency maps against clinicians through two main analyses - a direct comparison of saliency maps with the expert annotations of disease-specific pathologies and perturbation analyses using also expert annotations as saliency maps. We used a variety of explanation methods and obtained a comprehensive set of saliency maps for explaining the ensemble-based diagnostic decisions. In this study, we used two different network architectures and developed ensembles of DNNs to detect diabetic retinopathy and neovascular age-related macular degeneration from retinal fundus images and optical coherence tomography scans, respectively. To facilitate the adoption and success of such automated systems, however, it is crucial to validate saliency maps against clinicians. The quality of these maps are typically evaluated via perturbation analysis without experts involved. For imaging-based tasks, this is often achieved via saliency maps. To alleviate this issue, a range of explanation methods have been proposed to expose the inner workings of DNNs leading to their decisions.
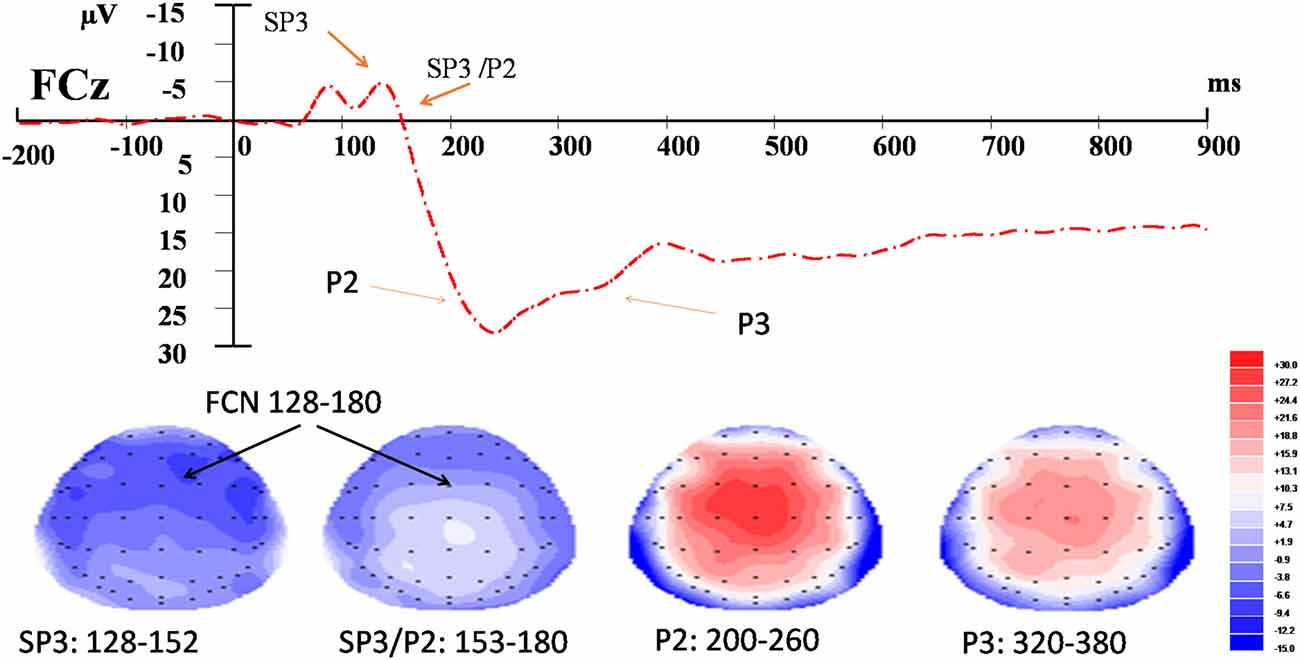
However, their decision mechanisms are often considered impenetrable leading to a lack of trust by clinicians and patients. Deep neural networks (DNNs) have achieved physician-level accuracy on many imaging-based medical diagnostic tasks, for example classification of retinal images in ophthalmology.
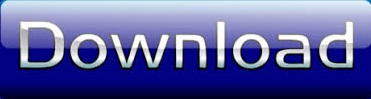